The good: This kind of data-driven strategy focuses on sourcing more financial and operational data and analyzing it quickly. That’s the magic that happens when you can access to a real data hub that incorporates financial data, but also granular operational data, which are the fire-starter of the revenue, cost, cash generation.
The bad: This kind of data-driven strategy is incomplete as it lacks vital data sources. It results in inaccurate data that becomes dangerous when used to craft strategies and make decisions. It generally happens when your financial system can sync and merge data from an old, siloed approach, but you still can't track relevant data or data that the proper granularity. E.g., Retailers who can't track hourly or day-of sales miss critical information that would inform cost-savings measures, like shift scheduling or sales generation, like in-store promotion.
The ugly: This kind of data-driven strategy can't get off the ground because its data is uninterpretable. Even if it could, finance would have no way to extract any value from its findings. If your financial and analysis (FP&A) system can tell you, for example, that you're going to sell more ice cream if the number of people in the city divided by the average salary of bakers in London is greater than the estimated CPI in three years. What do you do next? How do you derive a selling strategy from this accurate yet business agnostic finding that's pure math? Cool technology, but it lacks any actionable insight.
To help you determine where you land on the data-driven decision-making spectrum, I've made this handy chart to support you on your next steps.
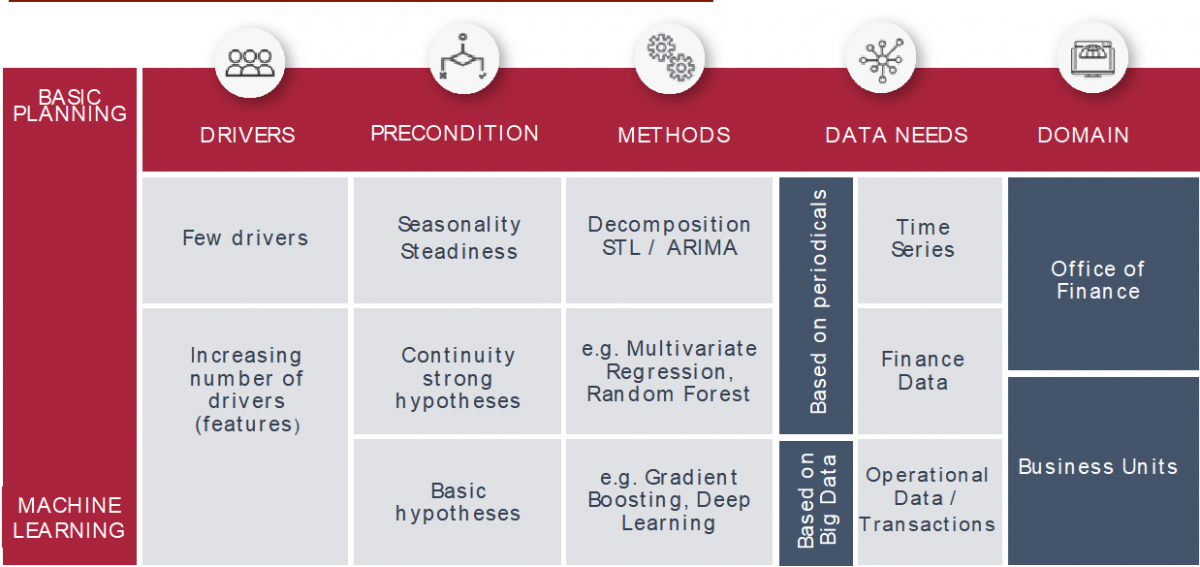
The crème de la crème of data-driven strategies is predictive analytics — specifically predictive analytics with explainable predictions. (I'll explain this concept in a bit.) Predictive analytics produces precise projections that can help shape decisions, guide course corrections, and redirect resources to productive activities.
In other words, a conscious and consistent journey towards predictive analytics will put you on the track towards — not just good — but exceptional data-driven decision making.
When executed correctly, predictive analytics has the power to leverage all kinds of data and confer predictive power on every financial process.
1. Understand the three essential pillars of predictive analytics
In the past, companies used external consultants and data scientists to build and utilize predictive functionality. The burdensome, costly nature of this approach still lingers in finance's imagination. Yet, times have changed. Although predictive technology has matured beyond recognition, there are several things that a predictive analytics solution must do:
- Unify data: Predictive platforms must facilitate a centralized approach to data management.
- Connect operational and financial data: By understanding the connection and interrelations between financial results and operational actions, you can better scrutinize and adjust operational strategy towards a scenario that would produce the optimal financial results.
- Be real-time: Access to real-time data is critical to producing precise predictions in times of uncertainty. In-memory computing and a powerful data engine are the two technologies that ensure real-time speed so you can gauge the impacts of unexpected market events or twists and turns in the economy and quickly determine a viable strategic response.
2. Use explainable predictions
Predictions are only half the battle when it comes to making data-driven decisions. The other half? Understanding what is driving your performance and impacting most the predicted outcomes.
For example, it’s helpful to know a product line’s predicted revenue. But it’s more beneficial to understand that your marketing campaigns and discount policy are the drivers of that revenue. This way, you could invest more in what's working, less in what's not and apply your insights to neighboring initiatives.
3. Use a suitable predictive analytics software
Leveraging a predictive solution without explainable predictions is, in my eyes, like providing a cart without a horse. It lacks a driving force. That’s why it’s important to recognize the main two types of predictive analytics software, as follows:
- Black box software: Black box software that gives you predictions but provides no rhyme or reason. You're expected to trust the machine's predictions without understanding the correlations it's made to come to its conclusion.
- Glass box approach software: This software produces the predictions and spots light on the business drivers responsible for them. This is supporting your savvy data-driven decision-making process because you can take those drivers, change the strategy, and simulate or re-shape the future towards a more fruitful outcome.
4. Don’t treat predictive analytics as a technology. Treat it as a solution
I've seen many organizations fall victim to shiny and new predictive analytics solutions that make data-driven decision-making more of an IT chore than a finance weapon. I suggest that, when you're vetting a predictive analytics solution or building your requirements for a data-driven strategy, be wary of these artificial intelligence (AI) and machine learning (ML) technology red flags:
- Highly tech but difficult to use
- AI/ML not integrated with FP&A tools: This leads to auditability problems and time-consuming manual processes.
- FP&A tools not integrated with ERP: Usually, ERP data models aren't meant for analysis. They're meant for transactional processes. It takes a lot of work to unearth ERP data that's beneficial for planning. Also, in the ERP world, data processes are transactional and fluid which impacts the data quality of snapshots.
5. Understand that you can start where you are today
Crawl, walk, run! Don't let perfect be the enemy of progress. Even as an end goal, predictive analytics becomes a baseline for improved automation, data synthesis, and the drive to underlie more predictive technologies under more processes — so it's ok to start lean and slow with what you have.
The point is: if you have even minimal data requirements and implement a predictive analytics software that includes explainable predictions, you'll still benefit from understanding performance drivers and automation, even if the predictions aren't 100% spot on.
Closing thoughts
We need to remember that our decisions are only as good as our data, and our data are only as good as the technology we use to understand and act on them.
When executed according to the framework and principles I've laid out for you here, the journey to predictive itself will result in data-driven decision-making based on data-accurate, data-efficient, and data-masterful financial processes.
*This article was originally published on FP&A Trends